Mustansar Abbas
- 502306
- Phd: 38th cycle
- Department of Veterinary Sciences
- Matriculation number: 1072830
- ORCID: orcid.org/0000-0003-3432-1016
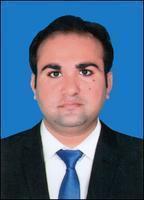
Phd thesis
Title: Artificial Intelligence (AI) applied through predictive models integrated into the livestock production process, towards sustainability and optimization of the sector development
Scientific background
In the past, farmers monitored the animals manually with the help of labor on the basis of physical appearance. These methods are not economical and have limitations ie, more time required, high labor cost, limited coverage of farms and human errors. To minimize these limitations, the use of sensors and other tools for the data collection on farms is growing, towards the development of the Precision Livestock Farming (PLF) approach. Precision Livestock Farming (PLF) is a system that utilizes advanced technologies, data analytics, and automated processes to monitor, manage, and optimize various aspects of livestock farming. It aims to improve animal health, welfare, productivity, and sustainability by providing farmers with real-time data and insights to make informed decisions and take proactive actions. PLF decreases labor availability, saving time on monitoring and increasing reliability. This approach to data collection and monitoring allows the adoption of data handling approaches from the industry and manufacturing sectors. In recent years, by increasing the population, demand for livestock products (milk, meat) has increased. This increasing trend of demand has increased the research on PLF (Garcia et al., 2020). By the advancement of technology ie, application of sensor on farms (eg, to measure feed and water intake), video recording cameras installation (to monitor individual animals and farms), different tools to measure environmental parameters (eg, air temperature, humidity, CO2, ammonia) and sound monitoring technology (eg, to monitor cough) (Hulbert, 2019) motivate stakeholders to invest more in PLF (CORDIS, 2022). Technology relies on substantial amounts of data to draw precise conclusions and generate accurate predictions about the growth pigs' health and production. However, the application of PLF in the field is still uncommon, and the different nature of the data collected by different sources and software in the farms make their connected interpretation difficult. Therefore, careful collection of data, data pre-processing and data validation processes are essential to make sure that technology will work efficiently and help farmers in the daily work (Tomas Norton, 2023).
Aims
- In collaboration with a private company of computer scientists, the study aims to develop a software able to collect farm data from different sources and of different nature in the same folder, allowing an easier data analysis and field application.
- Through the application of the tool in 22 commercial pig farms, a continuous data collection will be performed during a year to detect early signs of disease, stress, or discomfort in animals and develop predictive algorithms.
- The subsequent application in a subsample of pilot farms of the predictive models will support the farm managers in the decision process towards the improvement of the health and production of the farmed pigs.
Materials and methods
Creation of a platform for data collection from different sources: This section of the study will be carried out by a private company of computer scientists. They are developing a software that uses all the real time data from different sensors in the farms, stored in a cloud. They also are developing an application for mobile devices for the collection of daily sanitary data on farms by the personnel taking care of animals (e.g., mortality). Dashboards will be available for easy monitoring of the collected data.
Collection of data: To train the prediction model, data will be collected from twenty-two (22) different commercial pig farms during a year. Breeding, weaning and fattening sites will be included in the study (min = 300 pigs; max = 6000 pigs). We will collect data with the help of sensors, daily observations, laboratory sources and from slaughter-house.
Parameters collected from laboratory sources: Quality of feed by NIRS (Near infrared spectroscopy) analysis. To evaluate the health of pigs, different serological test will be performed on 30 pigs (n=30) of each batch i.e., PCR Polymerase Chain Reaction for the identification or detection of viral infection (PRRS, Porcine Reproductive and Respiratory Syndrome). PCR will be performed as per guideline provided by Wernike et al, 2012. In addition, information regarding possible new infectious diseases outbreaks will be collected. These parameters are manually updated daily on the cloud.
Parameters collected from the slaughter-house: The quality of carcasses and pathological lesions will also be collected. We will randomly select 100 pigs (n=100) from each batch to check the quality of carcasses. External, carcass quality will be checked with visual inspection (Lesion present or not on foreleg, hind leg, ear, tail). After slaughtering Internal carcass quality will be checked by dividing carcass into two parts, the “posterior” region, which included the hind legs and the tail, and the “anterior” region defined as the remaining area (starting from the loin up to the front limbs, the head, and the ears). Three 3-point scoring systems, defined by (Bottacini et al., 2018, Scollo et al., 2017) will be used. Score 0, up to one scratch or bite; Score 1, from two to five scratches or bites; Score 2, more than five scratches or bites or any wound which penetrates the muscle. All these readings will be saved to excel for further analysis.
Data analysis: After collection of data from different sources, all the information will be merged into a cloud and collected for statistical analysis. After data wrangling, basic statistical analysis will be done with suitable statistical software i.e., python, excel STAT or SPSS. Predicting models will be performed with the help of python software by using suitable predicting model i.e., Autoregressive Integrated Moving Average (ARIMA), Long Short-Term Memory (LSTM), Seasonal Autoregressive Integrated Moving Average (SARIMA) and Gaussian Processes (GPs). Excel STAT will be used for statistical process control. To guarantee an industrial approach to the handling of data from the pig sector, the data analysis will be supported by a collaboration with the Politecnico di Torino.
Parameters collected from the sensors: We will collect environmental parameters from the sensor i.e., temperature, humidity, and level of ammonia. Additionally, daily feed and water consumption will also be collected from the sensor. All the data collected from sensor will be stored daily at midnight in the cloud storage. At the beginning and at the end of the life cycle, weight of animals will be also collected for the feed conversion ratio (FCR) calculation.
Parameters collected from daily observations of farms’ personnel: With the help of trained workers, parameters like daily mortality, medicine use (antibiotics, anti-inflammatory), health observations and managerial interventions will be collected. Workers add these daily observations with the help of tablets. All the data collected from tablets will be stored daily at midnight in the cloud storage.
Application on the field: The developed tool will be applied to a subsample of farms (numerousness to be decided), to assist the farm managers in the decision-making process using predicting models and statistical process control.
Expected results.
- To fill the gap in existing tools to use large data sets from different sources and different nature in livestock.
- Artificial intelligence predictive models will help to optimize resources allocation, feed management, health, welfare, and productivity of the pigs.
- It will help to identify the diseases risk factors and predict diseases outbreaks, allowing an early intervention, with reduced losses and antibiotic consumption.
- This will enhance our decision-making ability on the base of real-time data and alarms, enhancing productivity and economical sustainability of the farm.
- Resources that are saved in the farms by optimizing environment and production will lead to other studies to investigate a possible reduced environmental impact of the commercial pig farming sector, also improving its environmental sustainability
Reference
CORDIS (2022). Website https://cordis.europa.eu. search term “precision livestock farming” Graduate Education and the Public Good (CGS) (2008). United States: Council of Graduate Schools.
Garcia, R., Aguilar, J., Toro, M., Pinto, A., & Rodriguez, P. (2020). A systematic literature review on the use of machine learning in precision livestock farming. Computers and Electronics in Agriculture, 179, 105826.
Hulbert, L. E. (2019). 5 Precision animal welfare for pigs. Journal of Animal Science, 97(Supplement_3), 11–12.
Thomas Norton. A practical approach on innovative tools to improve pig production. Proceedings of the 14th ESPHM, Thessaloniki 31st May to 2nd June 2023
Bottacini, M., Scollo, A., Edwards, S. A., Contiero, B., Veloci, M., Pace, V., & Gottardo, F. (2018). Skin lesion monitoring at slaughter on heavy pigs (170 kg): Welfare indicators and ham defects. PLoS One, 13(11), e0207115.
Scollo, A., Gottardo, F., Contiero, B., Mazzoni, C., Leneveu, P., & Edwards, S. A. (2017). Benchmarking of pluck lesions at slaughter as a health monitoring tool for pigs slaughtered at 170 kg (heavy pigs). Preventive veterinary medicine, 144, 20-28.
Wernike, K., Hoffmann, B., Dauber, M., Lange, E., Schirrmeier, H. and Beer, M., (2012).
Detection and typing of highly pathogenic porcine reproductive and respiratory syndrome virus by multiplex real-time rt-PCR. PLoS One, 7(6), p.e38251.
Research activities
Co supervisor
Alessandro Mannelli, Alberto Cavagnini
Publications/Research products:
- as a co-author (other than project)
1) Scollo Annalisa, Mustansar Abbas, Barbara Contiero, and Flaviana Gottardo. 2023. "Undocked Tails, Mycoplasma-like Lesions and Gastric Ulcers in Slaughtering Pigs: What Connection?" Animals 13, no. 2: 305. https://doi.org/10.3390/ani13020305
Current Activity:
The aim of the study is to develop a software in collaboration with a private company of computer scientists. After development, the software will be able to collect farm data from different sources and of a different nature in the same folder, allowing easier data analysis and field application. The software development requires big preliminary/raw data for training and for predictive modeling. So, currently we are collecting data from commercial pig farms.