Karina Brotto Rebuli
- SCIENZE VETERINARIE PER LA SALUTE ANIMALE E LA SICUREZZA ALIMENTARE
- Phd: 37th cycle
- Department of Veterinary Sciences
- Matriculation number: 975638
- ORCID: orcid.org/0000-0002-8606-0754
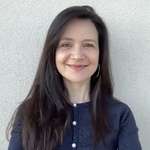
Phd thesis
Development of Machine Learning Models Applied to Milk Production of Holstein Friesian Cows in Automatic Milking Systems
My thesis explained in less than three minutes: Watch here.
This PhD project is part of the Circular Health for Industry (CH4I) project, whose general goals are (i) to study how to collect, manage and analyse data in a Circular Health approach without keeping separate data silos of human health, animals and plants and (ii) to develop Artificial Intelligence algorithms that work on them, to improve the infrastructure for collecting and analysing such data and to reskill workers for the adoption of AI technologies (https://ch4i.di.unito.it/). Within the CH4I, the work is being developed as part of the Subproject 2 Agrifood, specifically in the context of milk production of a herd that uses an Automatic Milking System (AMS) exclusively from 2016.
AMS, also called milking robots, generate a plenty of data on milk production and quality, milking behaviour and udder health, providing detailed information about each cow. This was not easily obtained with previous conventional systems and the extensive collection of data through the AMS has led to an exponentially growing amount of data [1]. However, very little has been done with this data to model milk production [2-6]. The goal of this PhD project is to develop methodologies and algorithms to fill this gap, particularly on milk production modelling with Machine Learning (ML) methods.
Currently, there are three branches of work in which the project is being developed:
(i) A clustering analysis with the milking data with the scope of characterising over lactation periods the groups of cows by their productivity. Preliminar results were discussed at the International Conference on Precision Dairy Farming, Vienna 2022. The article with final results, entitled Multi-algorithm clustering analysis for characterizing cow productivity on automatic milking systems over lactation periods [7], was publisehd at the Computers and Electronics in Agriculture journal.
(ii) Two models for prediction interval Genetic Programming were developed and will be applied on modelling milk production data. Preliminary results were presented at the Genetic and Evolutionary Computation Conference (GECCO), Boston 2022 [8] and the final versions of the models were presented at the XVI International Workshop on Artificial Life and Evolutionary Computation (WIVACE, Gaeta 2022 [9].
(iii) Interpretable ML model: The output of an interpretable model can be understood by humans. Thus, it has the potential for generating knowledge on the process that is being modelled. An Interpretable Genetic Programming model will be developed and applied on predicting the milk production of future lactation periods. The preliminary studies on this theme were presented in the poster A Comparison of Structural Complexity Metrics for Explainable Genetic Programming [10] at the Genetic and Evolutionary Computation Conference (GECCO), Lisbon 2023.
References:
[1] Jacobs, J. A., J. M. Siegford (2012). Invited review: The impact of automatic milking systems on dairy cow management, behavior, health, and welfare. Journal of Dairy Science, 95(5), 2227-2247.
[2] Masía, F. M., Lyons, N. A., Piccardi, M., Balzarini, M., Hovey, R. C., Garcia, S. C. (2020) Modeling variability of the lactation curves of cows in automated milking systems. Journal of Dairy Science, 103, 8189– 8196.
[3] Fuentes, S., Viejo, C. G., Cullen, B. , Tongson, E., Chauhan, S., S., Dunshea, F. R.(2020) Artificial Intelligence Applied to a Robotic Dairy Farm to Model Milk Productivity and Quality based on Cow Data and Daily Environmental Parameters. Sensors 2020, 20(10), 2975.
[4] Piwczynski, D., SitkowskaB., Aerts, J., Schork, P. M. 2020. Forecasting the milk yield of cows on farms equipped with automatic milking system with the use of decision trees. Animal Science Journal 2020, 91.
[5] Klis, P., Piwczynski, D., Sawa, A., Sitkowska, B. (2021). Prediction of Lactational Milk Yield of Cows Based on Data Recorded by AMS during the Periparturient Period. Animals 2021, 11, 383.
[6] Ji, B., Banhazi, T., Phillips, C. J.C., Wang, C., Li, B. (2022) A machine learning framework to predict the next month's daily milk yield, milk composition and milking frequency for cows in a robotic dairy farm. Biosystems Engineering, 216, 186–197.
[7] Rebuli, K. B., Ozella, L., Vanneschi, L., Giacobini M. (2023). Multi-algorithm clustering analysis for characterizing cow productivity on automatic milking systems over lactation periods, Computers and Electronics in Agriculture, Volume 211, 2023, 108002.
[8] Rebuli, K. B., Giacobini, M., Tallone, N., Vanneschi, V. (2022). A preliminary study of prediction interval methods with genetic programming. In Proceedings of the Genetic and Evolutionary Computation Conference Companion (GECCO '22). Association for Computing Machinery, New York, NY, USA, 530–533.
[9] Rebuli, K. B., Giacobini, M., Tallone, N., Vanneschi, L. (2023). Single and Multi-objective Genetic Programming Methods for Prediction Intervals. In: De Stefano, C., Fontanella, F., Vanneschi, L. (eds) Artificial Life and Evolutionary Computation. WIVACE 2022. Communications in Computer and Information Science, vol 1780. Springer, Cham.
[10] Rebuli, K. B., Giacobini, M., Silva, S., Vanneschi, V. (2023). A Comparison of Structural Complexity Metrics for Explainable Genetic Programming. In Proceedings of the Companion Conference on Genetic and Evolutionary Computation (GECCO '23 Companion). Association for Computing Machinery, New York, NY, USA, 539–542.
Research activities
Attended Congresses
- Brazilian Conference on Intelligent Systems, 25th to 29th September, 2023, Belo Horizonte, Brazil.
- GECCO 2023 (Genetic and Evolutionary Computation Conference), 15th to 19th July, 2023, Lisbon, Portugal. Link to the publication: https://dl.acm.org/doi/abs/10.1145/3583133.3590595
- EvoStar 2023 (European Conference on Genetic Programming, International Conference on the Applications of Evolutionary Computation, European Conference on Evolutionary Computation in Combinatorial Optimization and International Conference on Computational Intelligence in Music, Sound, Art and Design), 11th to 15th April, 2023, Brno, Czech Republic.
- WIVACE 2022 (XVI International Workshop on Artificial Life and Evolutionary Computation), 14th to 16th September, 2022, Gaeta, Italy. Link to the publication: https://link.springer.com/chapter/10.1007/978-3-031-31183-3_17
- 10th European Conference on Precision Livestock Farming (ECPLF 2022) and the 3rd International Conference on Precision Dairy Farming (PDC 2022), 29th August to 2nd September, Vienna, Austria.
- GECCO 2022 (The Genetic and Evolutionary Computation Conference), 9th to 13th July, 2022, Boston, United States. Link to the publication: https://dl.acm.org/doi/10.1145/3520304.3528806
- EvoStar 2022 (European Conference on Genetic Programming, International Conference on the Applications of Evolutionary Computation, European Conference on Evolutionary Computation in Combinatorial Optimization and International Conference on Computational Intelligence in Music, Sound, Art and Design), 20th to 22th April, 2022, Madrid, Spain.
- Game of Research - North Edition. 16h December, 2021. Turin, Italy.
Courses
- SPECIES Summer School on Evolutionary Computation, by SPECIES Society. Moraira, Spain. 2023.
- Summer School on Automatic Algorithm Design, by CRIStAL laboratory on the University of Lille's Cité Scientifique campus. University of Lille. 2023.
- Science Comunication and Public Engagement, by Prof. Daniel Edward Chamberlain, Prof. Gianpiero Vigani, and Prof. Enrico Caprio. University of Torino. 2023.
- Big Data and Digital Tools Applied to Livestock Production, by Prof. Guilherme J. M. Rosa and Prof. João Dorea. University of Padova. 2022.
- Grant Writing: How to write a Competitive Application to a Funding Agency, by Prof. Roger Coulombe. University of Torino. 2022.
- Bayesian Statistics for Genetics, by Prof. Ken Rice and Prof. Jonathan Wakefield. University of Washington. 2022.
- Complex Networks: Theory, Methods, and Applications, coord. Prof. Carlo Piccardi. Lake Como School of Advanced Studies. 2022.
- Filmaking for Scientists, by Prof. Samer Angelone. University of Torino. 2021.
Training periods abroad
- Computer Science Department at Federal University of Minas Gerais, Brazil. From 29th of Spetember to current, under the supervision of Prof. Dr. Gisele Pappa.
- Information Management School at NOVA University of Lisbon. From 20th of June 2023 to 2nd
of August 2023, under the supervision of Prof. Dr. Leonardo Vanneschi. - LASIGE Computer Science and Engineering Research Centre, Department of Informatics, Faculty of Sciences, University of Lisbon, Portugal. From 4th of October 2022 to 28th of February 2023, under the supervision of Prof. Dr. Sara Silva.
- Information Management School at NOVA University of Lisbon. From 4th of April 2022 to 4th of August 2022, under the supervision of Prof. Dr. Leonardo Vanneschi.